"OpenAI" has now become exactly what it was founded to oppose: another secretive large company building whatever they want while lobbying governments to prevent others from doing the same. They need competition.
Introducing...GPUTopia.
The war on open artificial intelligence is finally here. While many are enthusiastic and eager to dive in, a significant number are still grasping this shift's implications. This week, I talked with Christopher David, the founder of GPUTopia. If you're looking for a glimpse into the current landscape of this movement, check out the pod.
Today, we'll take a first look at what the GPUTopia's team has pioneered. But before that, let's unpack the broader landscape and its fascinating intersection with Bitcoin.
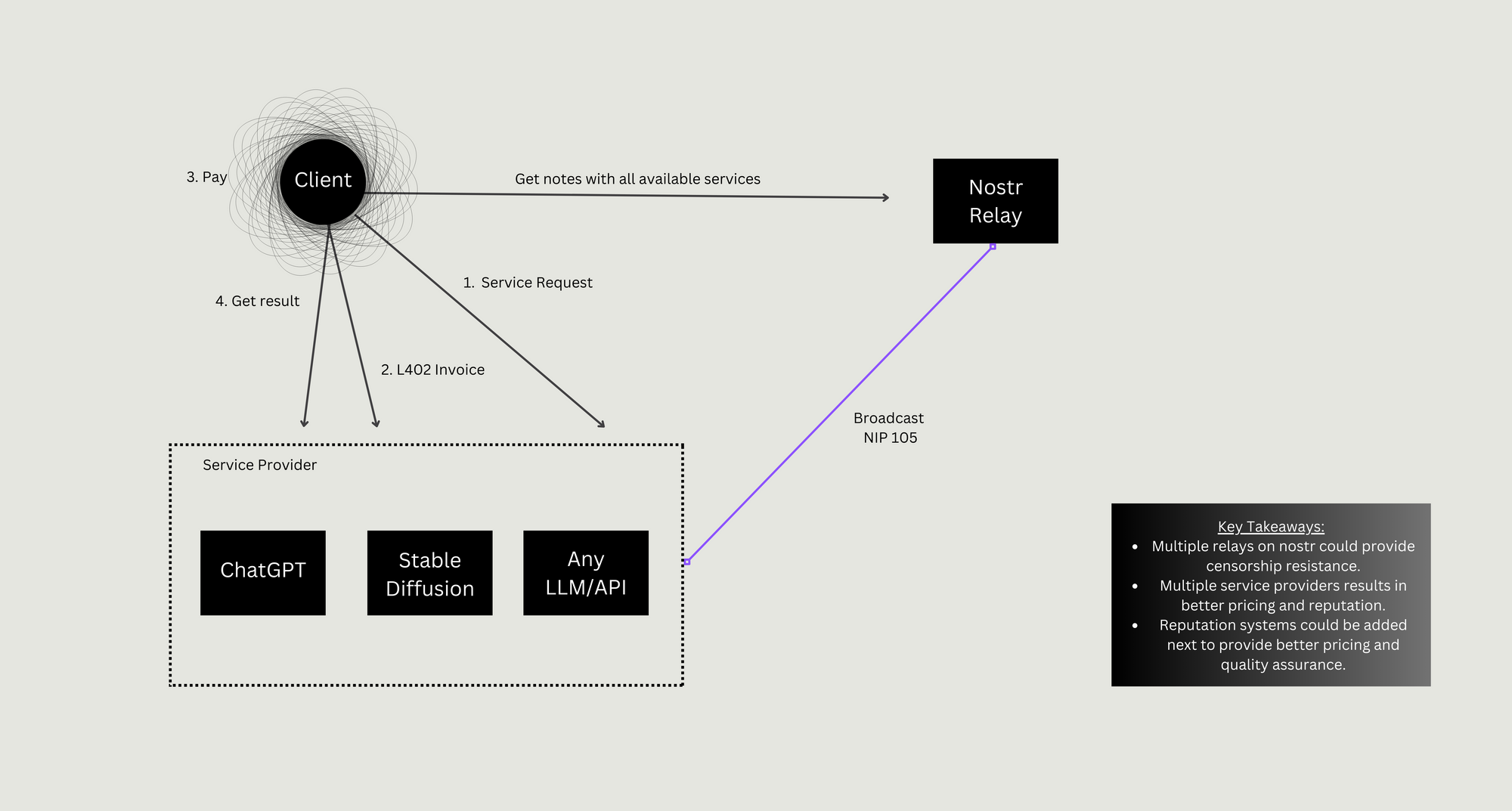
It is possible to run a simple service with about 300 lines of typescript and no front end. By doing it this way, we feel that we enable wider competition and thereby a race to the bottom on common commodity services. Why NIP-105 ?
Machine-to-machine is becoming increasingly popular in the builder space. By integrating the lightning network with open-source AI and combining various LLMs, there's tremendous potential. 👀 Peep "How (Actually) Open AI Wins" by Max of Hivemind Ventures for a good primer on the subject.
Banking the AIs
The real power of open source AI comes when you are able to combine a wide variety of openly trained and fine tuned models with other data and services: chaining them together and equipping them with tools to navigate the Internet and wider world. This was the general thesis behind Benchmark and Sequoia’s recent investment in LangChain, one of the fastest growing open source projects ever that makes it easy to link LLMs with each other and external data.
Because interacting with other agents and virtual resources has real world costs (e.g. compute, electricity, storage, bandwidth), these AI agents will soon need to interact economically – i.e. to exchange real world value for acquiring and performing services. I see no world where these machines are applying for accounts with JPM or Visa. Instead they’ll need a payment system that doesn’t care whether they’re a human or machine, that can integrate with existing payment systems, and that allows for global micropayments to pay for API calls to other machines.
What is the catalyst?
Over more than 10 years, Nvidia has built a nearly impregnable lead in producing chips that can perform complex A.I. tasks like image, facial and speech recognition, as well as generating text for chatbots like ChatGPT. The onetime industry upstart achieved that dominance by recognizing the A.I. trend early, tailoring its chips to those tasks and then developing key pieces of software that aid in A.I. development. Jensen Huang, Nvidia’s co-founder and chief executive, has since kept raising the bar. To maintain its leading position, his company has also offered customers access to specialized computers, computing services and other tools of their emerging trade. That has turned Nvidia, for all intents and purposes, into a one-stop shop for A.I. development.
Large enterprises like OpenAI, Microsoft, and mid-tier GPU cloud companies are racing to secure the most advanced AI hardware from NVIDIA. With a backlog spanning years, experts predict a pronounced supply constraint will persist.
However, a massive supply crunch at the market's pinnacle offers opportunities:
- Break NVIDIA's Monopoly with Software: NVIDIA's dominance is not just because of hardware but also its superior software. While AMD's hardware competes effectively with NVIDIA for AI tasks, NVIDIA's software supremacy gives them an edge. Yet, emerging projects, such as Tinygrad by George Hotz of CommaAI, aim to bridge the software gap for non-NVIDIA platforms, paving the way for additional capacity.
- Tapping into Unused GPU Capacity: Many powerful, newer AI models can run on consumer hardware. There's dormant GPU potential from previous crypto miners, especially from the Ethereum era before the Proof-of-Stake transition. With the advent of fractional micropayments, like those in Bitcoin, there's an opportunity to harness this idle capacity.
What's missing currently is an accessible marketplace. Researchers or startups without direct access to NVIDIA's resources or unwilling to commit to long-term contracts with cloud providers like Lambda need an on-demand compute source. The vision is a market where anyone can access the necessary compute power, even at a premium. In the long run, a balanced marketplace of this sort might drive down prices overall, challenging even the big cloud providers. The potential is in democratizing access, fostering innovation, and layering additional services atop this foundation.
GPUTopia my initial thoughts...
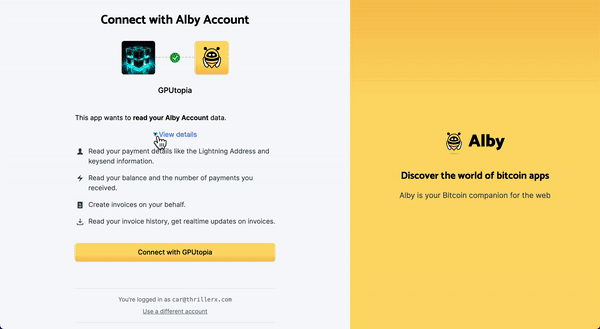
At the heart of GPUtopia's appeal is the Alby integration, which merges the convenience of a built-in Lightning wallet through a simple email/password login (integration gets GPUtopia's vision of enhancing social interactions and future nostr collaborations within its ecosystem.)
It is probable that future AI models might move beyond just increasing size. Instead, they might involve more intermediate steps, chaining smaller models, or engaging human intervention. This could highlight a potential need for human-driven, collaborative experiences in model training and innovation.
Interestingly enough, there is a growing need to think modularly. Whether integrating a model within another model or connecting an API with a model, the key lies in composability. This approach could be revolutionary, especially when coupled with a transparent social layer (see nostr) and driven by market forces (service marketplace.)
In essence, the future of AI, by GPUtopia, isn't just in creating bigger or better models. It lies in fostering a community-driven, modular, and collaborative ecosystem where innovations are not only possible but are also fairly compensated.
Thats an ai future we should be moving towards.